Bridging the Expertise Gap: Integrating LLMs and Knowledge Bases for Enterprise AI Mastery
Introduction:
As 2025 unfolds, large language models (LLMs) such as DeepSeek and ChatGPT continue to redefine natural language processing (NLP), offering unprecedented capabilities in text generation and comprehension. Their adoption spans industries, yet practical enterprise deployment faces persistent hurdles: factual inaccuracies, sector-specific knowledge gaps, opaque interpretability, and unreliable decision-support outputs. Compounding these challenges are the exorbitant computational costs of full-scale LLM deployment, particularly for firms prioritising on-premises solutions. This leaves organisations torn between financially prohibitive, high-parameter models and underwhelming “lightweight” alternatives—a dilemma that often culminates in abandoned AI projects yielding minimal commercial value.
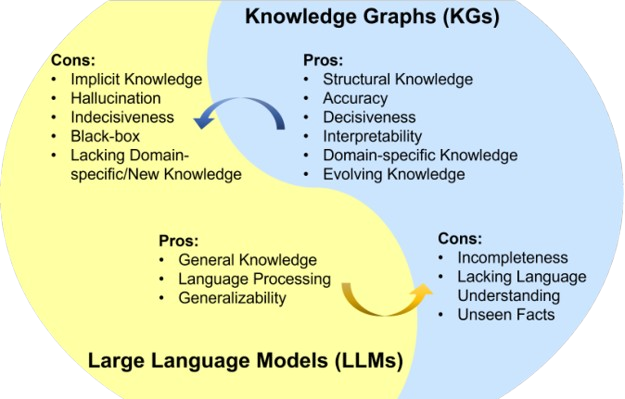
Synergising LLMs and Knowledge Bases: A Blueprint for Industrial-Grade AI
To address these limitations, enterprises are increasingly turning to hybrid architectures that marry LLMs with structured knowledge bases—a strategy that enhances both expertise and reliability.
1. Knowledge Bases as Factual Anchors
While LLMs excel at generalist language tasks, their propensity for “hallucinations” undermines credibility in specialised domains. Integrating curated knowledge bases—repositories of verified, industry-specific data—provides a critical corrective. In sectors such as manufacturing, maintenance, and quality assurance, these systems inject standardised best practices into AI outputs, ensuring recommendations are both accurate and actionable.
2. LLMs as Knowledge Base Accelerators
Traditional knowledge management relies on labour-intensive manual curation. LLMs disrupt this paradigm through automated data synthesis, entity recognition, and relational reasoning. By processing operational logs, production reports, and maintenance histories, they enable dynamic knowledge graph updates—slashing curation costs while maintaining rigour.
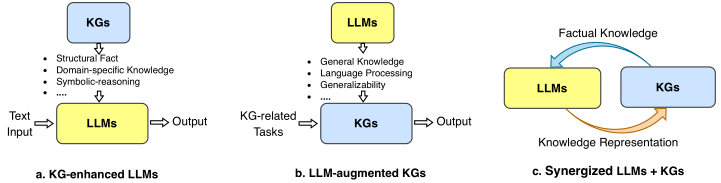
Architecting Industrial Intelligence: The LLM-Knowledge Base Feedback Loop
The most effective implementations create a symbiotic relationship: LLMs parse natural language queries, map them to structured knowledge graphs, and deliver auditable, logic-driven responses. This fusion proves particularly potent in predictive maintenance, supply chain optimisation, and process automation, where precision and explainability are paramount.
DPLUS: Realising Enterprise-Grade AI Through Data-Centric Engineering
While LLM-knowledge base integration shows promise, operationalising this approach demands robust data infrastructure. DPLUS—an industrial analytics and knowledge management platform—addresses this gap through three pillars:
1. Automated Knowledge Base Construction
- IoT-Driven Data Aggregation: Seamless integration with sensors, production systems, and operational databases ensures real-time, cleansed data pipelines.
- Context-Aware Modelling: Translates raw equipment metrics, process variables, and workflow logs into structured knowledge graphs.
- Cost-Efficient Deployment: Eliminates manual knowledge base development through AI-driven automation, accelerating ROI.
2. Delivering Trusted Industrial Intelligence
- Fact-Grounded AI Responses: Anchors LLM outputs to verified enterprise data, mitigating hallucination risks.
- Predictive Analytics Integration: Combines NLP-driven query resolution with prognostic insights for equipment health monitoring and process optimisation.
- Hybrid Cloud-Edge Architecture: Balances computational efficiency with data sovereignty requirements.
3. Optimising AI Economics
DPLUS enables firms to bypass the “large model trap”, instead deploying lean, sector-specific AI solutions that reduce training costs by up to 70% while maintaining performance.
From Chatbots to Corporate Strategists: Redefining AI’s Enterprise Role
With DPLUS, LLMs evolve from basic assistants into strategic partners:
✅Operational Embeddedness: Direct integration with production planning, quality control, and resource management systems.
✅Explainable Decision-Making: Auditable reasoning chains align AI outputs with regulatory and operational standards.
✅Scalable Expertise: Continuous knowledge graph refinement ensures AI remains abreast of evolving industrial practices.
The Path Forward: AI as a Core Competitive Asset
The 2025 enterprise landscape demands AI systems that blend linguistic sophistication with deep domain mastery. DPLUS exemplifies this shift, proving that value derives not from model size alone, but from tight integration with proprietary data and workflows.
As industries accelerate their digital transitions, the question for leadership teams is clear: will your organisation persist with underperforming AI experiments, or pivot to architectures that transform data into decisive advantage?
DPLUS: Engineering Intelligence, Delivering Value
In an era where AI maturity separates market leaders from laggards, DPLUS provides the infrastructure to harness LLMs not as costly curiosities, but as engines of operational and strategic transformation.
Is your enterprise prepared to bridge the AI execution gap?🤖💡
Explore how DPLUS can future-proof your decision-making capabilities.✨🚀
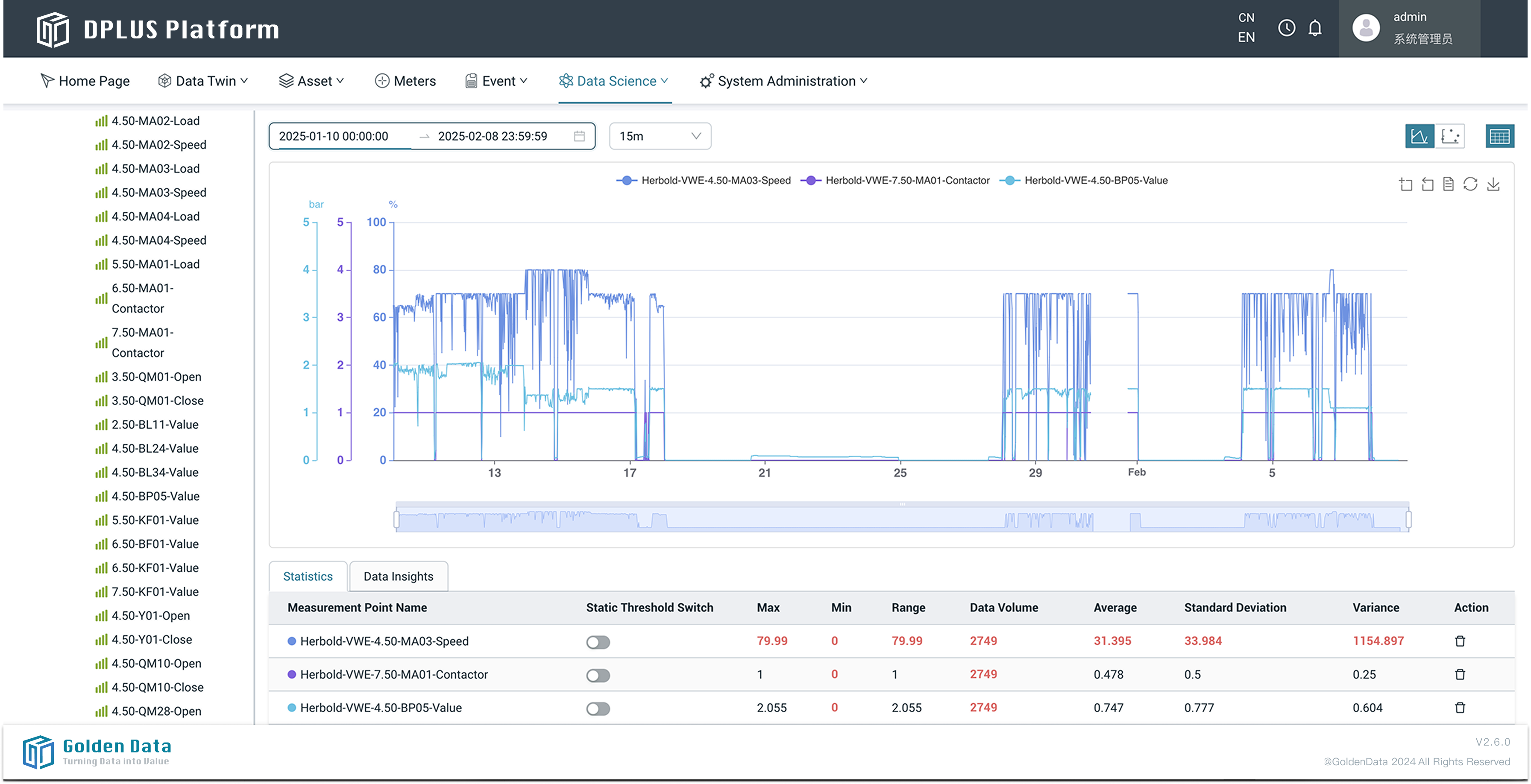
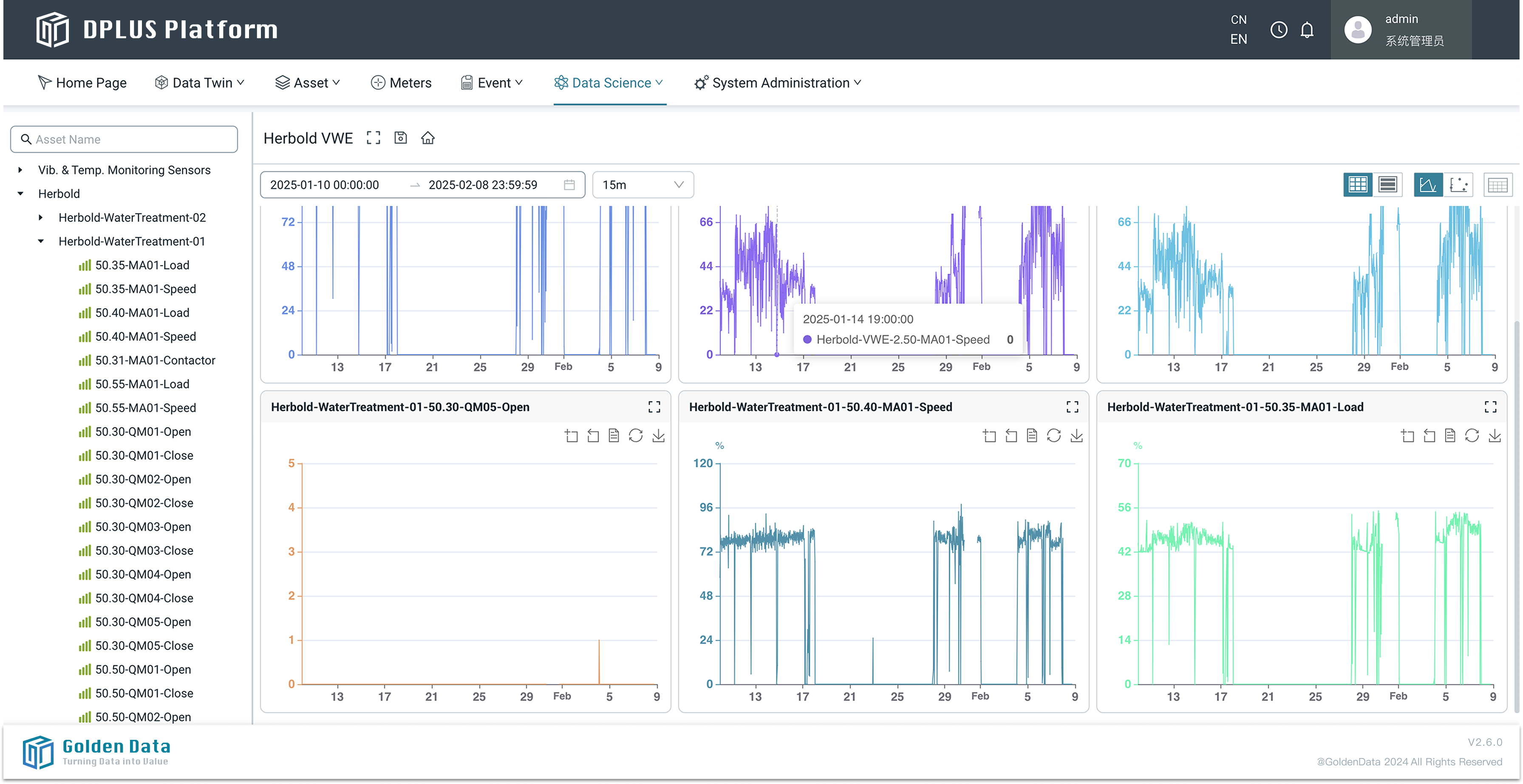
P.s.
Figures 1 and 2 in this article are cited from the original paper “Unifying Large Language Models and Knowledge Graphs: A Roadmap,” while Figures 3 and 4 are partial screenshots of the DPLUS Platform.
Choose Golden Data
To better understand, manage, and unlock the value behind data, Golden Data firmly believes that the forthcoming era of data and intelligence will revolutionize the traditional manufacturing industry's production methods and operational models.
Empower through Data
Data becomes an asset of a company whose value appreciates through not only data accumulation but data usage, and the new insights and value created for stakeholders of an organization in optimizing operation.
Work with Experts
Golden Data has a professional team, composed of experienced data experts from renowned universities. The experts team uses a wide range of methods in research and gives advice on data processing and modelling.
Rely on Tenacity
We love the thing we are doing and confident on our solutions. The team tries to eliminate distractions and obstacles in the face of adversity in each project.
Interested in our work?
Turning Data into Value