Smarter Fault Diagnosis in Industry: Merging Knowledge Graphs with Machine Learning
In the evolving landscape of industrial digitalisation, fault diagnosis is no longer confined to static rule sets or the intuition of veteran engineers. The combination of knowledge graphs (KGs) and machine learning (ML) is opening up new frontiers in how we monitor, detect, and prevent failures in critical equipment.
A recent innovation—outlined in the patent GB2619825A—demonstrates a transformational approach to industrial fault diagnosis. By integrating semantic knowledge networks with adaptive AI models, manufacturers can dramatically increase reliability, reduce maintenance costs, and uncover hidden operational insights.
🔍 From Raw Data to Intelligent Diagnosis
Traditional diagnostic systems rely on fixed rules or simple statistical thresholds. These can be rigid and fail to detect complex, evolving faults—especially in large, interdependent systems. The patented solution proposes a more intelligent method by combining:
- Knowledge Graphs: These represent the relationships between equipment, faults, causes, symptoms, and environmental factors. Imagine a digital “mind map” that helps the system reason through possible causes like a seasoned technician.
- Machine Learning Models: Once sensor data (vibration, temperature, pressure) is captured and processed, ML models are used to detect and classify faults with increasing accuracy over time.
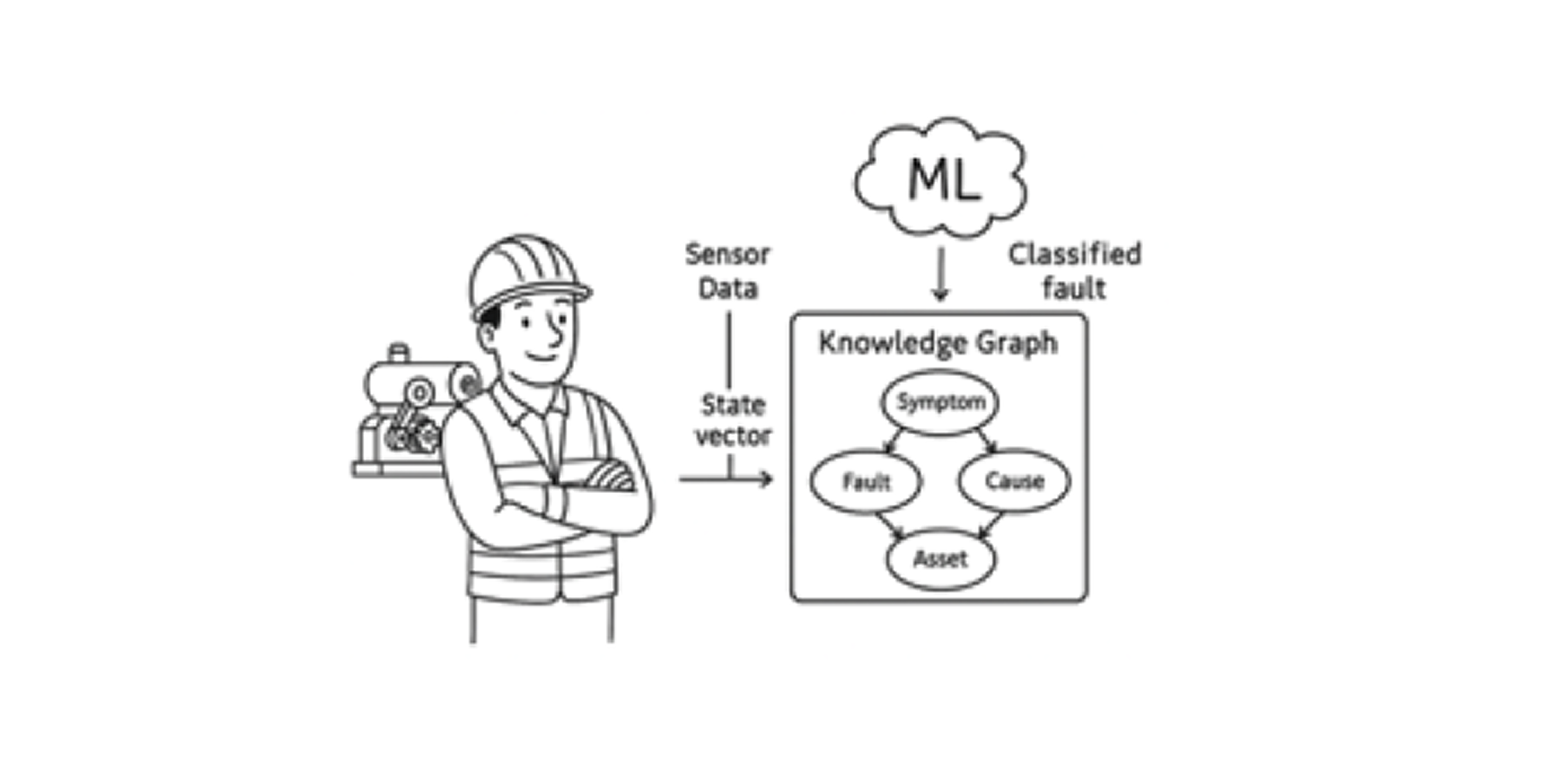
🧠 How the System Works
The method includes the following steps:
- Semantic Fault Mapping
A domain-specific knowledge graph is created, embedding fault types (e.g., bearing failure), symptoms (e.g., noise, heat), root causes (e.g., poor lubrication), and asset relationships. - Sensor Data Integration
Real-time sensor data is gathered and synchronised with the knowledge graph. Features like peak frequency, trend changes, and load behaviour are extracted. - AI-Powered Classification
ML algorithms such as neural networks or support vector machines classify fault types, often before they manifest physically. This proactive insight minimises unplanned downtime. - Feedback-Driven Learning
Each diagnosed fault updates the knowledge graph. Over time, the system becomes smarter, adjusting to new equipment behaviours or evolving fault modes.
🚀 Why It Matters
The convergence of semantic reasoning and data-driven modelling provides:
- Explainable AI: Maintenance teams can understand why a fault was predicted, not just what fault occurred.
- Adaptability: As new machines or fault types are introduced, the system evolves—requiring fewer manual updates.
- Faster Response: Automated early warnings mean failures can be prevented before they disrupt operations.
- Cross-System Intelligence: A shared knowledge graph allows diagnostic insights to transfer across equipment types and sites.
🔧 Applying It with DPLUS
To put this powerful methodology into practice, industrial teams need a platform capable of bridging data collection, AI analysis, and knowledge integration. That’s where the DPLUS system comes in.
DPLUS is an industrial-grade AI platform that provides:
- Real-time data ingestion from sensors, PLCs, and SCADA systems.
- A built-in AI engine for pattern recognition, anomaly detection, and predictive analytics.
- Digital twin modelling to map physical assets and integrate them with semantic fault frameworks.
- Tools to build and manage customised knowledge graphs that reflect each customer’s equipment, fault histories, and maintenance records.
By deploying DPLUS, businesses can turn raw operational data into structured, actionable intelligence, enabling the full implementation of the patent’s method. Whether you’re managing a cold rolling mill, a refinery, or a complex logistics network, DPLUS provides the infrastructure to automate fault detection, reduce downtime, and scale predictive maintenance across your operations.
📈 Ready to Transform Your Fault Diagnosis Strategy?
Contact us today to learn how DPLUS can help you build an intelligent, AI-powered maintenance ecosystem—driven by knowledge, backed by data, and designed for real-world impact.
About the Author
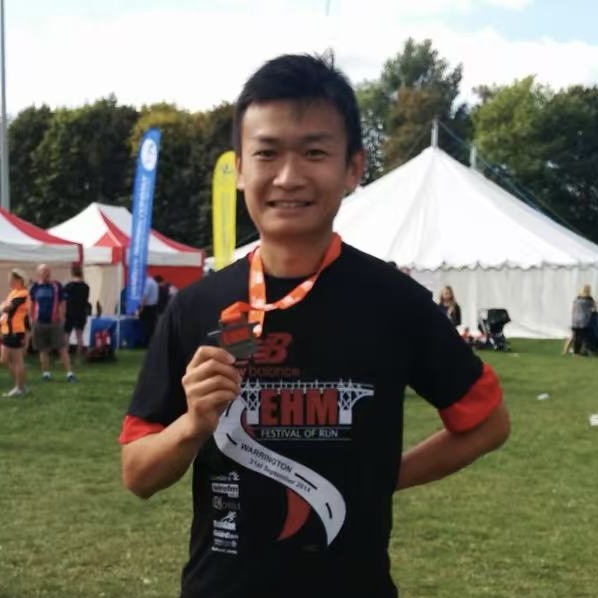
"Hi, I’m Dr Max Cao, I’m passionate about using AI and digital technologies to make industrial operations smarter, faster, and more sustainable. I love turning real-world challenges into practical solutions through innovation and collaboration. If you're interested in AI, digital twins, or industrial transformation, feel free to connect — I’d love to hear from you!"
Choose Golden Data
To better understand, manage, and unlock the value behind data, Golden Data firmly believes that the forthcoming era of data and intelligence will revolutionize the traditional manufacturing industry's production methods and operational models.
Empower through Data
Data becomes an asset of a company whose value appreciates through not only data accumulation but data usage, and the new insights and value created for stakeholders of an organization in optimizing operation.
Work with Experts
Golden Data has a professional team, composed of experienced data experts from renowned universities. The experts team uses a wide range of methods in research and gives advice on data processing and modelling.
Rely on Tenacity
We love the thing we are doing and confident on our solutions. The team tries to eliminate distractions and obstacles in the face of adversity in each project.
Interested in our work?
Turning Data into Value